Adversarial Examples to Test Explanation Robustness
PI: Leilani H. Gilpin
Assistant Professor of Computer Science and Engineering
University of California, Santa Cruz
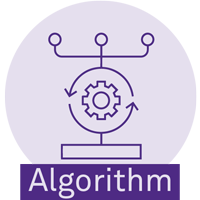
Framework component: Algorithm
A key component of pre-deployment verification of a machine learning agent is an explanation: a model-dependent reason or justification for the decision of the ML model. But ML model explanations are not standard nor do they have a common evaluation metric. Further, there is limited work on explaining errors or corner cases: current eXplainable AI (XAI) methods cannot explain why a prediction is wrong, or further why the model (may have) failed. In this project, we propose to develop a benchmark data set and testing protocol for XAI robustness. We will develop a data set for “stress testing” XAI methods that relies on new developments in adversarial machine learning and content generation.
Key Personnel
Oliver Chang
Graduate Student, Computer Science
University Of California, Santa Cruz
Shengjie Xu
Graduate Student, Electrical and Computer Engineering
University Of California, Santa Cruz
Outcomes and Updates
- A Framework for Generating Dangerous Scenes for Testing Robustness
-
Researchers Develop DANGER Framework for Stress Testing
-
"Explanation" is Not a Technical Term: The Problem of Ambiguity in XAI