A Practical Global Provenance System for Responsible Data Handling
PI: Michael Cafarella
Principal Research Scientist, Computer Science & Artificial Intelligence Laboratory
Massachusetts Institute of Technology
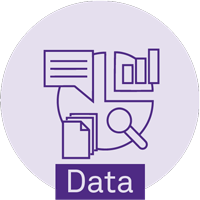
Framework component: Data
Many safety problems connected to machine intelligence require answering empirical questions about data handling; acting responsibly and safely requires that practitioners have information about how data was handled in the past. This provenance-style information is generally not collected and stored as a by-product of data activities, making it tedious and expensive to reconstruct at a later date. This information should be part of the daily routine of anyone engaged in machine intelligence work, but today using it is difficult and rare. Safe machine intelligence work requires a system for collecting and sharing data provenance that can be universally deployed across both tools and organizations. Universality means it must be designed to be far less expensive and obtrusive than past provenance attempts. Finally, it must be able to operate while still observing privacy and intellectual property concerns of its users. This project will construct three core software elements to develop an approach to universal data provenance collection in order to facilitate safe and responsible machine intelligence.
Key Personnel
Britt Youngmann
Postdoctoral Fellow, Computer Science & Artificial Intelligence Laboratory
Massachusetts Institute of Technology
Anna Zheng
Graduate Student, Computer Science
Massachusetts Institute of Technology