Incorporating Stability Objectives into the Design of Data-Intensive Pipelines
PI: Julia Stoyanovich
Institute Associate Professor of Computer Science & Engineering
Director, Center for Responsible AI, Tandon School of Engineering
Associate Professor of Data Science, Center for Data Science
New York University
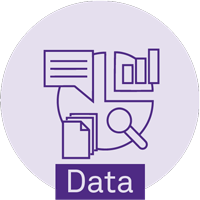
Framework component: Data
Stability is the property of an algorithmic system whereby small changes in the input lead to small changes in the output. Stability is a necessary (although not a sufficient) condition for reliability and trustworthiness of a system. Training data plays a central role in quantifying stability, and in intervening if stability lacks. In this project, we make two observations. The first is that training data is itself a product of complex multi-step data manipulation pipelines, in which data is integrated, cleaned, and otherwise preprocessed. The second observation is that data quality may be lower when it corresponds to members of historically disadvantaged groups, which may lead to lower predictive accuracy and stability for such groups. In this project, we will develop methods to quantify the impact of technical choices during data pre-processing on stability. We will also design interventions that improve model stability, both overall and for specific population groups.
Key Personnel
Andrew Bell
Graduate Student, Computer Science
New York University
Falaah Arif Khan
Graduate Student, Data Science
New York University
Lucas Rosenblatt
Graduate Student, Center for Responsible AI
New York University
Outcomes and Updates
- AI Ethics Debate at Chicago Conference, Precursor to CASMI’s Next Workshop
- Resilience in the Face of War: Ukrainian Researchers Investigate AI Fairness
-
Study Finds Machine Learning Technique May Worsen Fairness
- Automated Data Cleaning Can Hurt Fairness in Machine Learning-based Decision Making
-
An external stability audit framework to test the validity of personality prediction in AI hiring
- Github Repository for System Stability Audit Framework