Exploring Methods for Impact Quantification
PI: Ryan Jenkins
Associate Professor of Philosophy
California Polytechnic State University
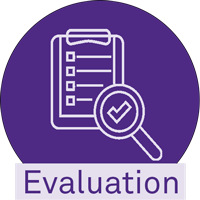
Framework component: Evaluation
While successful machine learning applications have proliferated in the last decade, there have also been storied failures, generating worries that ML applications can be biased or unfair, opaque or unaccountable. Understanding these impacts, prioritizing them, and assessing the success of practices in computer science to address them requires that we can assign precise and concrete values to the impacts of ML applications. However, there are currently no widely used, uncontroversial quantitative impact metrics (QIMs) for concretely measuring the human impacts of machine learning, a gap which this project seeks to remedy. Having such metrics in hand will help to: (1) ensure machine learning applications demonstrate the appropriate sensitivity to ethical concerns such as privacy, transparency, bias, and others; (2) prioritize interventions at various stages of the application development and deployment pipeline to achieve greatest leverage; and (3) compare the success of mitigation strategies, which will be essential for driving towards a set of empirically-grounded best practices in machine learning.
Key Personnel
Lorenzo Nericcio
Philosophy and Humanities Lecturer
California Polytechnic State University
Louisa Savageaux
Undergraduate Student, Philosophy and Economics
California Polytechnic State University
Roman Yampolskiy
Associate Professor of Computer Science and Engineering
University of Louisville
Outcomes and Updates
- Evaluating Metrics for Impact Quantification
- Researchers Pursue ‘Holy Grail’ to Measure Machine Learning’s Impact on People
-
Separating facts and evaluation: motivation, account, and learnings from a novel approach to evaluating the human impacts of machine learning